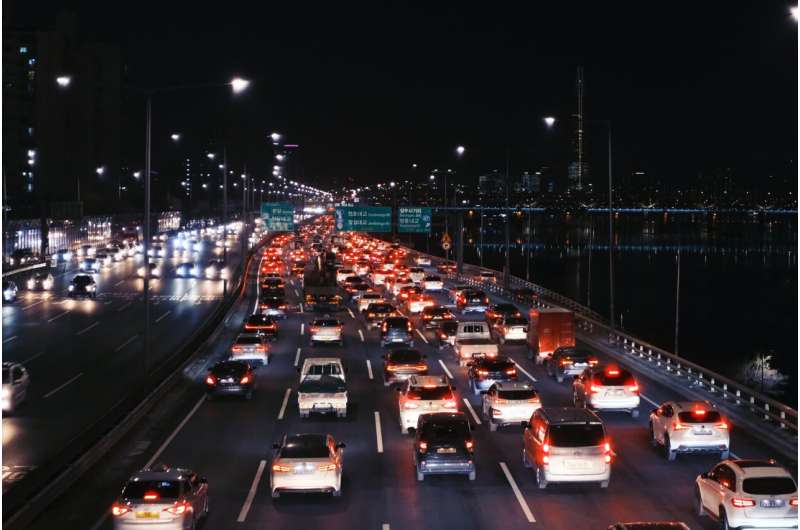
In the bustling corridors of the Penn Research in Embedded Computing and Integrated Systems Engineering (PRECISE) Center, groundbreaking research is unfolding.
Led by Nandan Tumu, an Electrical and Systems Engineering (ESE) doctoral student advised by Rahul Mangharam, Professor in the Departments of Computer and Information Science (CIS) and ESE, and PRECISE Center founding member, this work promises to transform urban traffic management, with implications for sustainable urban living and climate change mitigation.
A journey rooted in curiosity and innovation
Tumu's academic journey began at the University of Connecticut, where he majored in computer science and minored in philosophy. This unique combination laid a strong foundation for his research, blending technical expertise with a deep and nuanced understanding of uncertainty and knowledge.
His fascination with quantifying uncertainty and informing machine learning with physics grew from early endeavors in developing robotics control algorithms using reinforcement learning.
A major roadblock in today's approach to machine learning is sample complexity, which is the question of how much data is needed for learning algorithms to achieve the right level of performance. The more data, the more energy required, and the greater the impact on the environment.
To address this, Tumu explored more efficient methods and discovered that physics-informed and constrained learning could significantly reduce the need for extensive sampling
By integrating this approach with conformal prediction, a method for distribution-free uncertainty quantification, Tumu found a way to control complex systems efficiently and reliably.
This innovative pairing of physics-informed and constrained learning and conformal prediction has become the driving force of his research, promising to unlock the potential of larger multi-agent systems, such as fleets of drones or driverless cars, or infrastructure like power grids and wind farms.
Transforming urban traffic with differentiable predictive control
Optimizing transportation systems has been a motivating application for Tumu's research. In 2023, he joined a team at Pacific Northwest National Labs (PNNL) as a summer intern to develop machine learning methods for traffic system control.
In their paper, "Differentiable Predictive Control for Large-Scale Urban Road Networks," published on the arXiv preprint server, Tumu and his collaborators address one of the most pressing issues of our time: traffic congestion and its contribution to CO2 emissions. Since transportation is a major driver of global emissions, optimizing traffic networks is essential for reducing energy consumption and mitigating climate change.
Tumu's novel approach leverages Differentiable Predictive Control (DPC), a physics-informed machine learning methodology developed at PNNL, to advance traffic management. Most existing traffic-control systems rely on some flavor of Model Predictive Control (MPC), which typically breaks down road networks into regions and then predicts and optimizes the traffic flow in each region.
In contrast to MPC, which can scale poorly and require significant time to solve traffic flow problems, Tumu found that DPC can solve those problems accurately and quickly, offering a more robust solution to traffic management.
Indeed, empirical comparisons with existing state-of-the-art Model Predictive Control (MPC) methods demonstrate the superiority of Tumu's approach.
As reported in the paper, DPC leads to a four-order-of-magnitude reduction in computation time and up to a 37% improvement in traffic performance. Additionally, the controller's robustness to scenario shifts ensures adaptability to changing traffic patterns. This work not only proposes more efficient traffic control methods, but also aims to reduce emissions and alleviate congestion in large-scale urban networks.
Real-world impact and future directions
The practical implications of Tumu's research will be evaluated through PNNL's collaboration with the city of Coral Gables, Florida, as a part of the AutonomIA project. The goal is to implement these advanced traffic control algorithms—strategies for managing traffic lights and signals—in a real-world setting, in order to significantly reduce travel time and energy consumption.
The results so far are promising: the project estimates a substantial reduction in vehicle delays, contributing to lower overall energy consumption and a decrease in CO2 emissions.
"This innovative approach to optimizing existing traffic control infrastructure is a crucial step in the fight against climate change," says Ján Drgoňa, Research Data Scientist and one of Tumu's mentors at PNNL.
Tumu's research extends beyond urban traffic. In collaboration with PNNL, he is applying and advancing DPC methodologies to enhance the efficiency of existing wind farms. "This extension aligns with my overarching research vision of developing control algorithms for networked cyber-physical systems to enhance efficiency and performance," Tumu says.
"By incorporating physics-based information and uncertainty quantification, I aim to create improved control algorithms that leverage real-world data."
A vision for a sustainable future
"Nandan Tumu's research embodies mathematically rigorous and scalable approaches to addressing critical climate and complex societal challenges," says Mangharam.
"By integrating physics-informed machine learning with advanced control methodologies, he is pioneering solutions that promise to make our urban environments more efficient and our energy systems more sustainable."
"His work testifies to the power of interdisciplinary research and its potential to drive meaningful change in our world," adds PRECISE Center Director Insup Lee, Cecilia Fitler Moore Professor in CIS.
"As Nandan continues to push the boundaries of what is possible, his contributions are set to leave a lasting impact on both academia and society."
More information: Renukanandan Tumu et al, Differentiable Predictive Control for Large-Scale Urban Road Networks, arXiv (2024). DOI: 10.48550/arxiv.2406.10433
Journal information: arXiv
Citation: Pioneering efficient traffic control and sustainable energy solutions (2024, September 13) retrieved 13 September 2024 from https://techxplore.com/news/2024-09-efficient-traffic-sustainable-energy-solutions.html
This document is subject to copyright. Apart from any fair dealing for the purpose of private study or research, no part may be reproduced without the written permission. The content is provided for information purposes only.