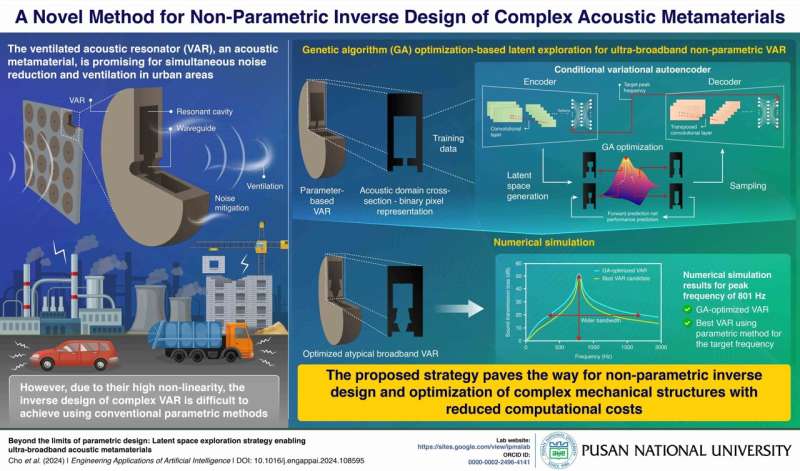
Noise pollution has become increasingly common in urban areas, stemming from traffic, construction activities, and factories, which can seriously impact health, causing stress, sleep disturbances, and cardiovascular issues. Consequently, various methods for noise reduction have been proposed, such as physically blocking the path of sound and active noise control. However, since sound travels through air, physically blocking sound can also lead to poor ventilation, highlighting the need for research into simultaneous sound attenuation and ventilation.
Acoustic metamaterials (AMs) have been extensively studied as a promising solution for this purpose owing to their unique acoustic properties. Recently, a new type of AM, called a ventilated acoustic resonator (VAR), has been proposed that can manipulate both sound waves and airflow using only geometric shapes. It can block even low-frequency noise with a compact structure while maintaining ventilation.
A VAR consists of a waveguide that guides sound waves to a resonant cavity that traps them. For appropriate performance, a VAR requires a functional shape optimized for broadband sound attenuation across a target peak frequency. However, conventional analytical design methods only allow relatively simple parametric designs and cannot be used for achieving VARs with complex geometries.
To address this limitation, a team of researchers from Korea, led by Associate Professor Sang Min Park from the School of Mechanical Engineering at Pusan National University developed an innovative deep-learning-based inverse design method.
"We proposed a latent-space exploration strategy that searches for broadband VAR with the target frequency through genetic algorithm-based optimization. Compared to conventional methods, our approach allows for high design flexibility while reducing computational costs," explains Dr. Park.
Their study was made available online on May 15, 2024, and published in the journal Engineering Applications of Artificial Intelligence.
In the proposed inverse design method, a conditional variational autoencoder (CVAE), a deep-learning generative model, encodes the geometric features of the VAR in the latent space. The latent space is a lower-dimensional space that contains the essential information of a higher-dimensional input, in this case, the VAR.
To generate this space, the CVAE is trained with cross-section images of the resonant cavity of VAR and peak frequency information. The generated latent space is then used for genetic algorithm (GA) optimization, aimed at searching for a VAR with broadband sound attenuation performance for various peak target frequencies. GA applies a natural-selection-based approach to search for optimized VAR over multiple successive generations, much like the selection of favorable genes in biological evolution.
The researchers trained the CVAE with cross-section images of VAR with a T-shaped resonant cavity with varying values for its design parameters. Using this data, their optimization strategy produced a non-parametric VAR with an atypical but functional structure.
The researchers compared the optimization results with the VAR having the widest bandwidth in the training data for each target frequency and found that the optimized designs exhibited broader bandwidths in all cases. Furthermore, they compared the performance of the non-parametric VAR to that designed using a parameter-based inverse design method and found that the former had considerably larger bandwidths.
Highlighting the significance of these results, Dr. Park says, "Our ultra-broadband VARs can be deployed in urban environments to effectively reduce noise pollution without compromising ventilation, thereby improving quality of life by creating quieter, more comfortable living and working spaces.
"Additionally, our strategy opens new horizons for artificial-intelligence-based design of complex mechanical structures, potentially revolutionizing fields like automotive and aerospace engineering."
This pioneering design method represents a significant step towards the AI-driven design of AMs and other complex mechanical structures.
More information: Min Woo Cho et al, Beyond the limits of parametric design: Latent space exploration strategy enabling ultra-broadband acoustic metamaterials, Engineering Applications of Artificial Intelligence (2024). DOI: 10.1016/j.engappai.2024.108595
Citation: Researchers use AI to create powerful sound-dampening materials (2024, August 8) retrieved 8 August 2024 from https://techxplore.com/news/2024-08-ai-powerful-dampening-materials.html
This document is subject to copyright. Apart from any fair dealing for the purpose of private study or research, no part may be reproduced without the written permission. The content is provided for information purposes only.