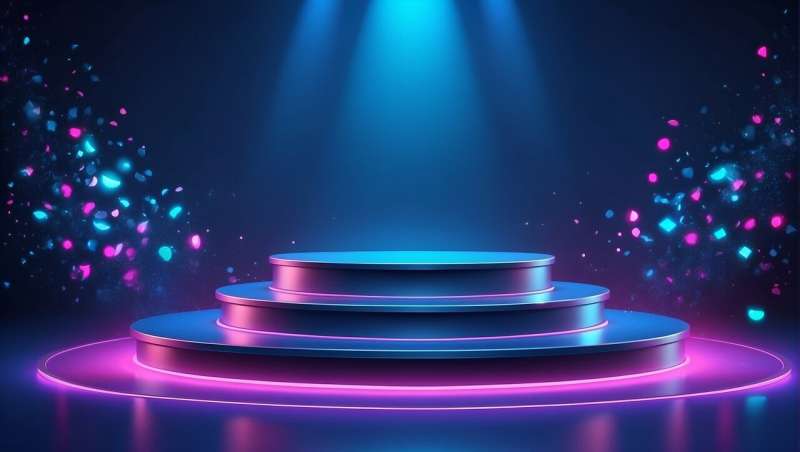
Whether it's the Oscars, the Tonys, or the Grammys, observers annually make predictions as to which actor, film, musical, or song will win these coveted awards—with forecasts based on what experts say impresses the voters. "Grammy voters love to give Record of the Year to a carefully crafted throwback jam," the Los Angeles Times wrote ahead of this year's Grammy Awards.
A team of New York University researchers has systematized this process by creating an algorithm that takes into account a song's traits, such as its lyrics, along with other information, including Billboard rankings, to illuminate the variables of successful songs—specifically, those voted as winners for Song of the Year, Record of the Year, and Rap Song of the Year in 2021, 2022, and 2023. In doing so, the work goes beyond some previous methods by not only making predictions, but also by identifying the traits of Grammy winners.
"Spotting award-winning art is surely a subjective process and is complicated by the secrecy surrounding voters' decisions," says Anasse Bari, a clinical associate professor at New York University's Courant Institute of Mathematical Science and the senior author of the study, which appears on IEEE Xplore. "However, by taking into account what we know about the songs themselves—from their make-up to their popularity—we can pinpoint those likely to be celebrated.
"We think this AI tool could help to identify emerging artists and trends by unearthing music that is likely to be popular—and that otherwise might go undiscovered."
In constructing the AI tool, the researchers created a dataset of nominees from 2004 to 2020 across three award categories—Song of the Year, Record of the Year, and Rap Song of the Year—totaling nearly 250 songs. They then combined a range of variables and trained AI algorithms to learn from these historical data, which included Billboard rankings and Google search volume (how frequently users searched for a nominated song in the year it was nominated).
The algorithm also took into account a song's musical characteristics, using Spotify data deployed by previous studies, which included the following:
- Acousticness: Whether or not the track is acoustic (i.e., reliant on non-electric instruments or sounds)
- Danceability: How suitable a track is for dancing
- Energy: A perceptual measure of intensity and activity
- Instrumentalness: A measure of the lack of vocals in a track
- Speechiness: The presence of spoken words in a track
Finally, the AI tool included a song's lyrics, using commonly deployed Natural Language Processing algorithms to capture words and the sentiments these words conveyed. The calculations revealed a song's vocabulary diversity, its emotional tone (e.g., happy, sad, angry), and even profane language.
The researchers then determined if the resulting algorithm could generate a list of likely winners by identifying top three candidates drawn from all of the nominees for Song of the Year, Record of the Year, and Rap Song of the Year in each year of the studied period (2021-2023)—a total of 27 songs from among approximately 75 nominees.
The results showed that the model accurately included all nine winning songs across the three categories in its top three list—among them, Billie Eilish's "everything i wanted" (2021 Record of the Year), Silk Sonic's "Leave the Door Open" (2022 Song of the Year), and Kendrick Lamar's "The Heart Part 5" (2023 Rap Song of the Year).
The authors add that some of the model's predictions ran counter to those made by betting sites. For instance, Bonnie Raitt's "Just Like That," which the model placed in its top three for 2023 Song of the Year, was seen as one the songs least likely to win that year by gambling platforms. In addition, H.E.R."s Grammy-winning "I Can't Breathe," which the model placed in its top three for 2021 Song of the Year, was viewed as a long shot by betting sites.
Interestingly, the predictive features varied among the categories. For Song of the Year, the most predictive features included energy, acousticness, and the peak Billboard position of the song. By contrast, for Record of the Year, the most predictive features included speechiness, profanity, and acousticness. For Rap Song of the Year, the most predictive features included vocabulary diversity, the number of words, and the happiness score.
While the study's authors caution that the algorithm is not a precise prediction tool that forecasts winners, it can nonetheless surface wide-ranging attributes associated with successful tunes.
"Our findings highlight the importance of considering multiple factors, such as popularity and music specific features, when predicting the winners of music awards," says Bari, who leads the Courant Institute's Predictive Analytics and AI Research Lab.
"More broadly, the work shows the potential of using machine learning and data-driven techniques to gain insights into the factors that contribute to a song's success."
The study's other authors were members of Bari's AI research group in NYU's Department of Computer Science: Rushabh Musthyala, Abhishek Narayanan, and Anirudh Nistala.
More information: Rushabh Musthyala et al, An AI Framework for Predicting the Winner of the Grammys, 2024 9th International Conference on Big Data Analytics (ICBDA) (2024). DOI: 10.1109/ICBDA61153.2024.10607237
Citation: AI tool challenges betting sites with Grammy predictions (2024, July 31) retrieved 31 July 2024 from https://techxplore.com/news/2024-07-ai-tool-sites-grammy.html
This document is subject to copyright. Apart from any fair dealing for the purpose of private study or research, no part may be reproduced without the written permission. The content is provided for information purposes only.