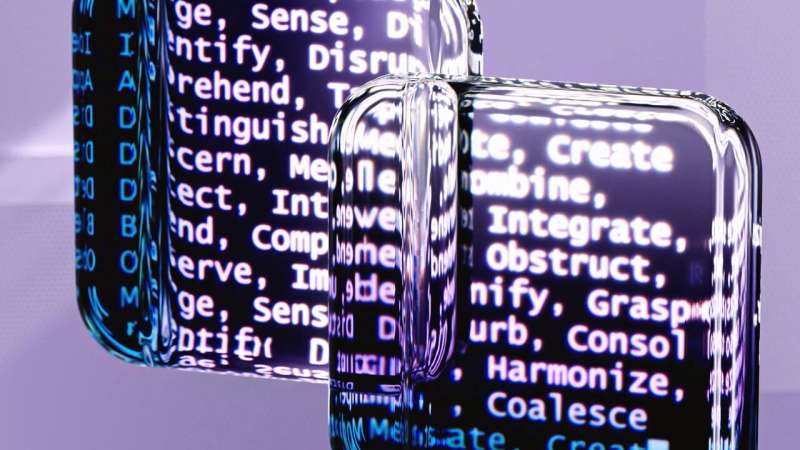
Inspired by the map makers of the 20th Century, Imperial researchers have demonstrated a new way to identify copyright holders' work in LLMs.
The technique was presented at the International Conference on Machine Learning in Vienna this week, and is detailed in this preprint on the arXiv server.
Generative AI is taking the world by storm, already transforming the day-to-day lives of millions of people.
Yet today, AI is often built on "shaky" legal grounds when it comes to training data. Modern AI models, such as Large Language Models (LLMs), require vast amounts of text, images and other forms of content from the internet to achieve its impressive capabilities.
In a new paper from Imperial College London experts, researchers propose a mechanism to detect the use of data for AI training.
They hope that their proposed method will serve as a step towards greater openness and transparency in a rapidly evolving field of Generative AI, and will help authors better understand how their texts are used.
Lead researcher Dr. Yves-Alexandre de Montjoye, from Imperial's Department of Computing, said, "Taking inspiration from the map makers of the early 20th century, who put phantom towns on their maps to detect illicit copies, we study how injection of 'copyright traps'—unique fictitious sentences—into the original text enables content detectability in a trained LLM."
First, the content owner would repeat a copyright trap multiple times across their collection of documents (e.g. news articles). Then, if an LLM developer scrapes the data and trains a model on it, the data owner would be able to confidently prove training by observing irregularities in the model's outputs.
The proposal is best suited for online publishers, who could hide the copyright trap sentence across news article, such that it stays invisible to the reader, yet is likely to be picked up by a data scraper.
However, Dr. de Montjoye emphasizes how LLM developers could develop techniques to remove traps and avoid detection. With traps being embedded in several different ways across news articles, successfully removing all of them is likely to require significant engineering resources to stay ahead of new ways to embed them.
To verify the validity of the approach, they partnered with a team in France, training a "truly bilingual" English-French 1.3B-parameter LLM, injecting various copyright traps into the training set of a real-world state-of-the-art parameter-efficient language model. The researchers believe the success of their experiments enables better transparency tools for the field of LLM training.
Co-author Igor Shilov, also from Imperial College London's Department of Computing, added, "AI companies are increasingly reluctant to share any information about their training data. While the training data composition for GPT-3 and LLaMA (older models released by OpenAI and Meta AI respectively) is publicly known, it is no longer the case for the more recent models GPT-4 and LLaMA-2.
"LLM developers have little incentive to be open about their training procedure, leading to a concerning lack of transparency (and thus fair profit sharing), making it more important than ever to have tools to inspect what went into the training process."
Co-author Matthieu Meeus, also from Imperial College London's Department of Computing, said, "We believe the issue of AI training transparency and discussions on fair compensation for content creators to be very important for the future where AI is built in a responsible way. Our hope is that this work on copyright traps contributes towards a sustainable solution."
More information: Matthieu Meeus et al, Copyright Traps for Large Language Models, arXiv (2024). DOI: 10.48550/arxiv.2402.09363
Journal information: arXiv
Citation: Phantom data could show copyright holders if their work is in AI training data (2024, July 29) retrieved 29 July 2024 from https://techxplore.com/news/2024-07-phantom-copyright-holders-ai.html
This document is subject to copyright. Apart from any fair dealing for the purpose of private study or research, no part may be reproduced without the written permission. The content is provided for information purposes only.