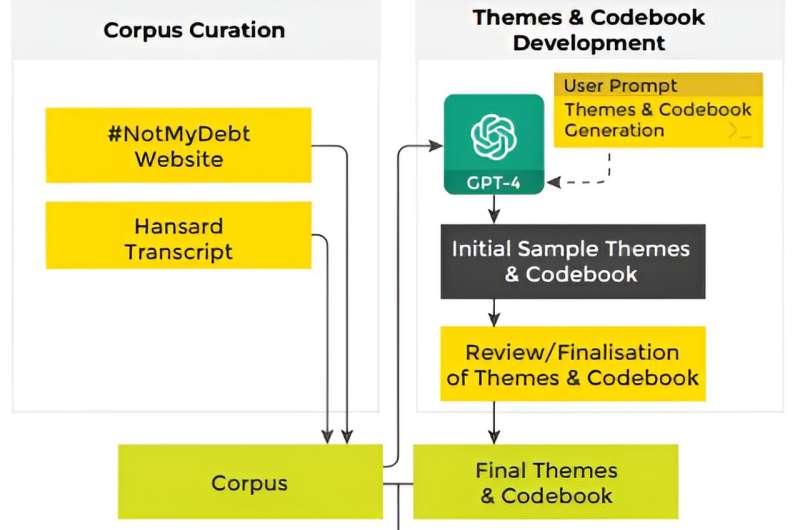
In a pilot study, posted to the arXiv preprint server, researchers have found evidence that large language models (LLMs) have the ability to analyze controversial topics such as the Australian Robodebt scandal in similar ways to humans—and sometimes exhibit similar biases.
The study found that LLM agents (GPT-4 and Llama 2 ) could be prompted to align their coding results with human assignments, through thoughtful instructions: "Be Skeptical!" or "Be Parsimonious!" At the same time, LLMs can also help identify oversights and potential analytical blind spots for human researchers.
LLMs are promising analytical tools. They can augment human philosophical, cognitive and reasoning abilities, and support "sensemaking"—making sense of a complex environment or subject—by analyzing large volumes of data with a sensitivity to context and nuance absent in earlier text processing systems.
The research was led by Dr. Awais Hameed Khan from the University of Queensland node of the ARC Center of Excellence for Automated Decision-Making & Society (ADM+S).
"We argue that LLMs should be used to assist—and not replace—human interpretation.
"Our research provides a methodological blueprint for how humans can leverage the power of LLMs as iterative and dialogical, analytical tools to support reflexivity in LLM-aided thematic analysis. We contribute novel insights to existing research on using automation in qualitative research methods," said Dr. Khan.
"We also introduce a novel design toolkit—the AI Sub Zero Bias cards, for researchers and practitioners to further interrogate and explore LLMs as analytical tools."
The AI Sub Zero Bias cards help users structure prompts and interrogate bias in outputs of generative AI tools such as Large Language Models. The toolkit comprises of 58 cards across categories relating to structure, consequences and output.
Drawing on creativity principles, these provocations explore how reformatting and reframing the generated outputs into alternative structures can facilitate reflexive thinking.
This research was conducted by ARC Center of Excellence for Automated Decision-Making and Society (ADM+S) researchers Dr. Awais Hameed Khan, Hiruni Kegalle, Rhea D'Silva, Ned Watt, Daniel Whelan -Shamy, under the guidance of Dr. Lida Ghahremanlou, Microsoft Research, and Associate Professor Liam Magee, from the ADM+S node at Western Sydney University.
This research group began their collaboration at the 2023 ADM+S Hackathon where they developed the winning project Sub-Zero. A Comparative Thematic Analysis Experiment ofRobodebt Discourse Using Humans and LLMs.
Associate Professor Liam Magee has been mentoring the group since first meeting them at the Hackathon.
"The ADM+S Hackathon was instrumental in bringing together these researchers from across multiple disciplines and universities," said Associate Professor Magee.
"The research has been a tremendous group contribution, and I'd like to acknowledge both the efforts of the team and the logistical support of Sally Storey and ADM+S in making this possible."
More information: Awais Hameed Khan et al, Automating Thematic Analysis: How LLMs Analyse Controversial Topics, arXiv (2024). DOI: 10.48550/arxiv.2405.06919
Journal information: arXiv
Provided by ARC Centre of Excellence for Automated Decision-Making and Society
Citation: Study: Large language models are biased, but can still help analyze complex data (2024, July 17) retrieved 17 July 2024 from https://techxplore.com/news/2024-07-large-language-biased-complex.html
This document is subject to copyright. Apart from any fair dealing for the purpose of private study or research, no part may be reproduced without the written permission. The content is provided for information purposes only.