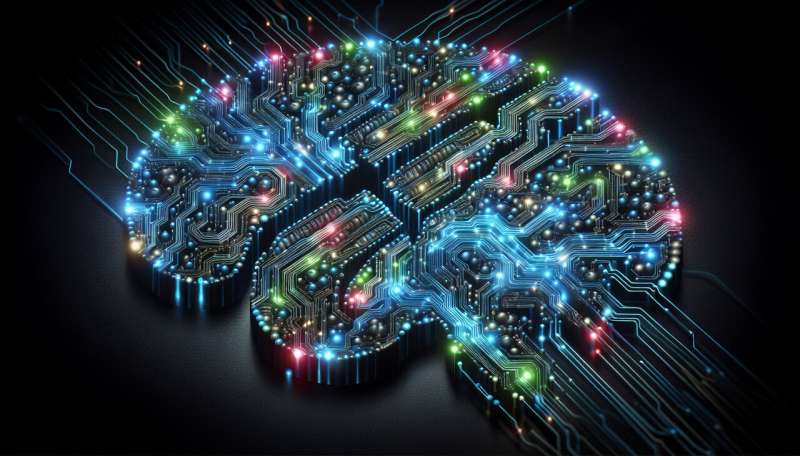
Computers have come so far in terms of their power and potential, rivaling and even eclipsing human brains in their ability to store and crunch data, make predictions and communicate. But there is one domain where human brains continue to dominate: energy efficiency.
"The most efficient computers are still approximately four orders of magnitude—that's 10,000 times—higher in energy requirements compared to the human brain for specific tasks such as image processing and recognition, although they outperform the brain in tasks like mathematical calculations," said UC Santa Barbara electrical and computer engineering Professor Kaustav Banerjee, a world expert in the realm of nanoelectronics.
"Making computers more energy efficient is crucial because the worldwide energy consumption by on-chip electronics stands at #4 in the global rankings of nation-wise energy consumption, and it is increasing exponentially each year, fueled by applications such as artificial intelligence."
Additionally, he said, the problem of energy inefficient computing is particularly pressing in the context of global warming, "highlighting the urgent need to develop more energy-efficient computing technologies."
Neuromorphic (NM) computing has emerged as a promising way to bridge the energy efficiency gap. By mimicking the structure and operations of the human brain, where processing occurs in parallel across an array of low power-consuming neurons, it may be possible to approach brain-like energy efficiency.
In a paper published in the journal Nature Communications, Banerjee and co-workers Arnab Pal, Zichun Chai, Junkai Jiang and Wei Cao, in collaboration with researchers Vivek De and Mike Davies from Intel Labs propose such an ultra-energy efficient platform, using 2D transition metal dichalcogenide (TMD)-based tunnel-field-effect transistors (TFETs).
Their platform, the researchers say, can bring the energy requirements to within two orders of magnitude (about 100 times) with respect to the human brain.
Leakage currents and subthreshold swing
The concept of neuromorphic computing has been around for decades, though the research around it has intensified only relatively recently. Advances in circuitry that enable smaller, denser arrays of transistors, and therefore more processing and functionality for less power consumption are just scratching the surface of what can be done to enable brain-inspired computing.
Add to that an appetite generated by its many potential applications, such as AI and the Internet-of-Things, and it's clear that expanding the options for a hardware platform for neuromorphic computing must be addressed in order to move forward.
Enter the team's 2D tunnel-transistors. Emerging out of Banerjee's longstanding research efforts to develop high-performance, low-power consumption transistors to meet the growing hunger for processing without a matching increase in power requirement, these atomically thin, nanoscale transistors are responsive at low voltages, and as the foundation of the researchers' NM platform, can mimic the highly energy efficient operations of the human brain.
In addition to lower off-state currents, the 2D TFETs also have a low subthreshold swing (SS), a parameter that describes how effectively a transistor can switch from off to on. According to Banerjee, a lower SS means a lower operating voltage, and faster and more efficient switching.
More information: Arnab Pal et al, An ultra energy-efficient hardware platform for neuromorphic computing enabled by 2D-TMD tunnel-FETs, Nature Communications (2024). DOI: 10.1038/s41467-024-46397-3
Citation: Researchers propose the next platform for brain-inspired computing (2024, June 26) retrieved 26 June 2024 from https://techxplore.com/news/2024-06-platform-brain.html
This document is subject to copyright. Apart from any fair dealing for the purpose of private study or research, no part may be reproduced without the written permission. The content is provided for information purposes only.