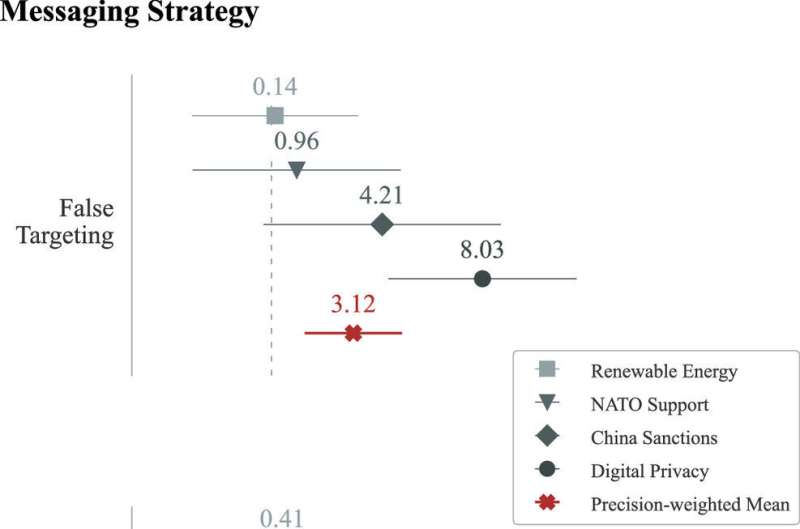
Recent advancements in large language models (LLMs) have raised the prospect of scalable, automated, and fine-grained political microtargeting on a scale previously unseen. For example, when integrated with existing databases of personal data, LLMs could tailor messages to appeal to the vulnerabilities and values of specific individuals, such that a 28 year-old, non-religious, liberal female with a college degree receives a very different message than a 61 year-old, religious, conservative male who only graduated from high school.
In their new article, "Evaluating the persuasive influence of political microtargeting with large language models," published in PNAS, the OII's doctoral researcher Kobi Hackenburg and Professor Helen Margetts address this possibility, investigating the extent to which access to this individual-level data increases the persuasive influence of OpenAI's GPT-4 model.
By building a custom web application capable of integrating demographic and political data into GPT-4 prompts in real time, the OII researchers generated thousands of unique messages tailored to persuade individual users.
Deploying this application in a pre-registered, randomized control experiment, they found that messages generated by GPT-4 were broadly persuasive. Crucially, however, in aggregate, the persuasive impact of microtargeted messages was not statistically different from that of non-microtargeted messages.
This suggests—contrary to widespread speculation—that the influence of current LLMs may reside not in their ability to tailor messages to individuals, but rather in the persuasiveness of their generic, non-targeted messages.
Kobi Hackenburg said, "There are really two plausible explanations for this result: either text-based microtargeting is itself not a very effective messaging strategy, or GPT-4 is simply unable to microtarget effectively when deployed in a manner similar to our experimental design.
"We already know, for example, that even current frontier LLMs cannot always reliably reflect the opinion distributions of fine-grained demographic groups; a capability which would seem necessary for accurate microtargeting."
Professor Helen Margetts added, "This finding is important because the leaders of big tech firms have made grand claims about the persuasiveness of microtargeting by LLMs like GPT. This research has gone some way towards challenging such claims. In contrast, it highlights the persuasiveness of GPT's 'best message' on specific policy issues, without targeting."
More information: Kobi Hackenburg et al, Evaluating the persuasive influence of political microtargeting with large language models, Proceedings of the National Academy of Sciences (2024). DOI: 10.1073/pnas.2403116121
Citation: Effectiveness of large language models in political microtargeting assessed in new study (2024, June 26) retrieved 26 June 2024 from https://techxplore.com/news/2024-06-effectiveness-large-language-political-microtargeting.html
This document is subject to copyright. Apart from any fair dealing for the purpose of private study or research, no part may be reproduced without the written permission. The content is provided for information purposes only.