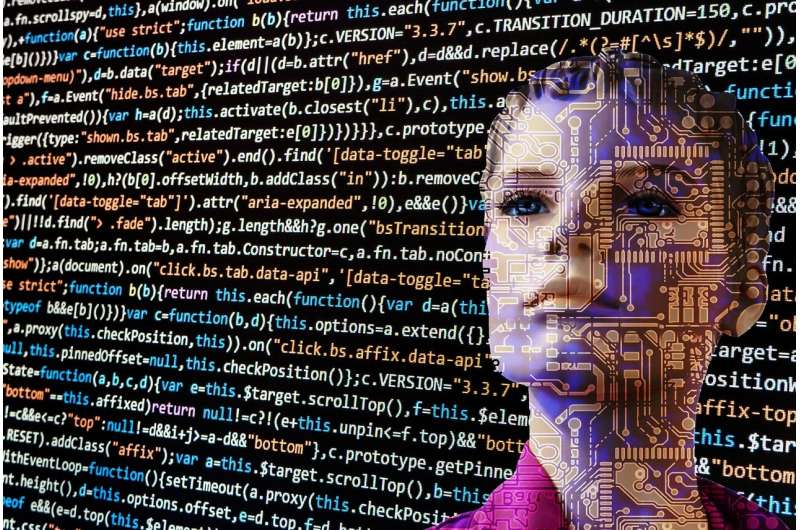
By now, all of us have heard and read a lot about artificial intelligence (AI). You've likely used some of the countless AI tools that are becoming available. For some, AI feels like a magic wand that predicts the future.
But AI is not perfect. A supermarket meal planner in Aotearoa New Zealand gave customers poisonous recipes, a New York City chatbot advised people to break the law, and Google's AI Overview is telling people to eat rocks.
At its core, an AI tool is a particular system that addresses a particular problem. With any AI system, we should match our expectations to its abilities—and many of those come down to how the AI was built.
Let's explore some inherent shortcomings of AI systems.
Trouble in the real world
One of the inherent issues of all AI systems is that they are not 100% accurate in real-world settings. For example, a predictive AI system will be trained using data points from the past.
If the AI then comes across something new—not similar to anything in the training data—it most likely won't be able to make the correct decision.
As a hypothetical example, let's take a military plane equipped with an AI-powered autopilot system. This system will function thanks to its training "knowledge base." But an AI really isn't a magic wand, it's just mathematical computations. An adversary could create obstacles the plane AI cannot "see" because they are not in the training data, leading to potentially catastrophic consequences.
Unfortunately, there is not much we can do about this problem, apart from trying to train the AI for all possible circumstances that we know of. This can sometimes be an insurmountable task.
Bias in the training data
You may have heard about AI making biased decisions. Usually, bias happens when we have unbalanced data. In simple terms, this means that when training the AI system, we are showing it too many examples of one type of outcome and very few of another type.
Let's take the example of an AI system trained to predict the likelihood a given individual will commit a crime. If the crime data used for training the system mostly contains people from group A (say, a particular ethnicity) and very few from group B, the system won't learn about both groups equally.
As a result, its predictions for group A will make it seem these people are more likely to commit crimes compared to people from group B. If the system is used uncritically, the presence of this bias can have severe ethical consequences.
Thankfully, developers can address this issue by "balancing" the data set. This can involve different approaches, including the use of synthetic data—computer-generated, pre-labeled data built for testing and training AI that has checks built into it to protect against bias.
Being out of date
Another issue with AI can arise when it's been trained "offline" and isn't up to date with the dynamics of the problem it is meant to work on.
This article is republished from The Conversation under a Creative Commons license. Read the original article.
Citation: Opinion: AI is not a magic wand—it has built-in problems that are difficult to fix and can be dangerous (2024, June 17) retrieved 17 June 2024 from https://techxplore.com/news/2024-06-opinion-ai-magic-wand-built.html
This document is subject to copyright. Apart from any fair dealing for the purpose of private study or research, no part may be reproduced without the written permission. The content is provided for information purposes only.