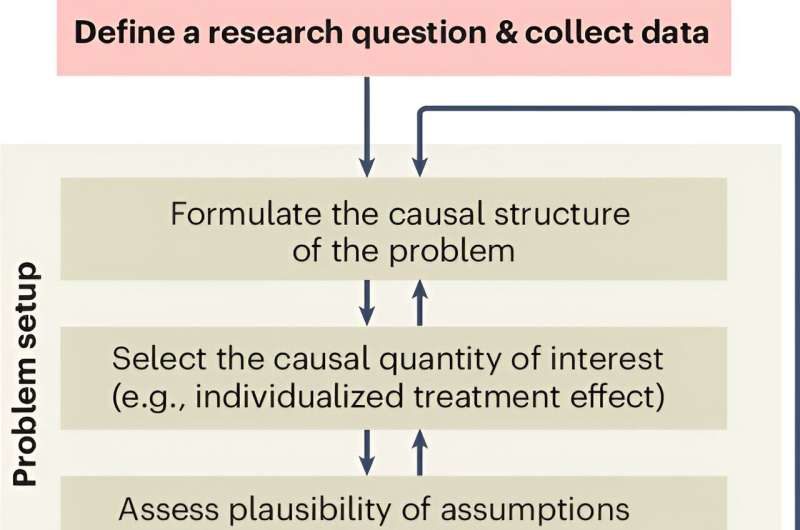
Machines can learn not only to make predictions, but to handle causal relationships. An international research team shows how this could make medical treatments safer, more efficient, and more personalized.
Artificial intelligence techniques can be helpful for multiple medical applications, such as radiology or oncology, where the ability to recognize patterns in large volumes of data is vital. For these types of applications, the AI compares information against learned examples, draws conclusions, and makes extrapolations.
Now, an international team led by researchers from Ludwig-Maximilians-Universität München (LMU) and including researchers from the University of Cambridge, is exploring the potential of a comparatively new branch of AI for diagnostics and therapy.
The researchers found that causal machine learning (ML) can estimate treatment outcomes—and do so better than the machine learning methods generally used to date. Causal machine learning makes it easier for clinicians to personalize treatment strategies, which individually improves the health of patients.
The results, reported in the journal Nature Medicine, suggest how causal machine learning could improve the effectiveness and safety of a variety of medical treatments.
Classical machine learning recognizes patterns and discovers correlations. However, the principle of cause and effect remains closed to machines as a rule; they cannot address the question of why. When making therapy decisions for a patient, the 'why' is vital to achieve the best outcomes.
"Developing machine learning tools to address 'why?' and 'what if?' questions is empowering for clinicians, because it can strengthen their decision-making processes," said senior author Professor Mihaela van der Schaar, Director of the Cambridge Center for AI in Medicine. "But this sort of machine learning is far more complex than assessing personalized risk."
For example, when attempting to determine therapy decisions for someone at risk of developing diabetes, classical ML would aim to predict how probable it is for a given patient with a range of risk factors to develop the disease.
With causal ML, it would be possible to answer how the risk changes if the patient receives an anti-diabetes drug; that is, gauge the effect of a cause. It would also be possible to estimate whether metformin, the commonly-prescribed medication, would be the best treatment, or whether another treatment plan would be better.
To be able to estimate the effect of a hypothetical treatment, the AI models must learn to answer "what if?" questions. "We give the machine rules for recognizing the causal structure and correctly formalizing the problem," said Professor Stefan Feuerriegel from LMU, who led the research. "Then the machine has to learn to recognize the effects of interventions and understand, so to speak, how real-life consequences are mirrored in the data that has been fed into the computers."
More information: Stefan Feuerriegel et al, Causal machine learning for predicting treatment outcomes, Nature Medicine (2024). DOI: 10.1038/s41591-024-02902-1
Citation: Training AI models to answer 'what if?' questions could improve medical treatments (2024, June 14) retrieved 14 June 2024 from https://techxplore.com/news/2024-06-ai-medical-treatments.html
This document is subject to copyright. Apart from any fair dealing for the purpose of private study or research, no part may be reproduced without the written permission. The content is provided for information purposes only.