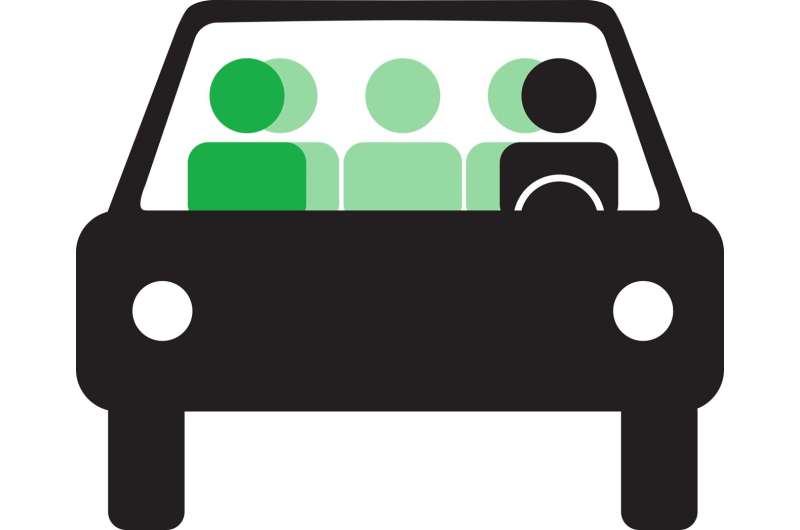
Imagine hailing a dynamic shuttle whenever you need to go somewhere—and arriving faster than if you had just driven yourself. That's the vision shared by Berkeley researchers: a world where environmentally friendly, energy-efficient high-occupancy vehicles (HOVs) are the preferred and quickest mode of transportation.
Using a simulated environment, the researchers tested a novel traffic signal control algorithm that works to maximize the throughput of people—rather than vehicles—at intersections. Dubbed HumanLight, the technology uses reinforcement learning, a type of artificial intelligence, to prioritize and reward passengers of HOVs with more green lights. Their findings, published in Transportation Research Part C, showed that the resultant travel time savings creates a strong incentive for people to choose transit options over single-occupancy cars.
The study's lead author is Dimitris Vlachogiannis, Ph.D. Co-authors are Scott Moura, the Clare and Hsieh Wen Shen Distinguished Professor in Civil and Environmental Engineering; principal investigator Jane Macfarlane, director of the Smart Cities Research Center; and Hua Wei, assistant professor at Arizona State University.
Moura and Macfarlane recently spoke with Berkeley Engineering about this work, explaining how it could someday provide a more democratic and sustainable traffic management solution.
How did your previous work lead you to HumanLight?
Jane: I helped launch OnStar, and we had seat sensors, much like those used with air bags, that would tell us how many people were in the car in the event of an emergency or accident. [I thought] if we have a way of knowing how many people are sitting in those vehicles waiting at a traffic signal, we can develop a traffic signal control system, like HumanLight, that gives priority to those with higher occupancy.
Scott: We had this seven-year project called NEXTCAR, where we looked at ways to optimize the speed of the vehicle to reduce energy consumption based on the traffic light timing. As I got deeper into it, I started to wonder, what if we could control traffic light timing? Transportation engineers, meanwhile, were thinking, but how do you control traffic light timing if the vehicle flow is uncontrollable? But what if we could control both?
Jane and Dimitris then introduced the idea of HumanLight, which looks at how to control traffic light timing so that we can maximize the throughput of people, not cars—which is ultimately what matters.
Why should people care about traffic management?
Scott: The simple answer is: 51 billion tons. That's the amount of greenhouse gases emitted annually around the world. A little less than 1% of that is the state of California. And 40% of California's greenhouse gas emissions are from transportation. Today, California is focused on bringing that toward zero to address the challenges of climate change.
One way to meet that goal is to have everybody buy EVs. But that would require building a whole new supply chain and shifting the world economy. In the meantime, another option is to go after the low-hanging fruit. Ultimately, we're talking about software and using all the hardware and infrastructure that we already have—but being more strategic about how we manage traffic light timing to reduce congestion and energy consumption.
Jane: At an infrastructure level, when you start thinking about who's controlling traffic, you realize that we have a controlled chaotic mess out there. There are city traffic engineers who tune traffic lights and set up the timing. Then you have the Department of Transportation managing freeway on-ramps and off-ramps. In addition, there are numerous navigation apps that are moving people through our city and sometimes directing cars into residential neighborhoods not designed for lots of traffic.
We're getting more people on the roads every year, so we need to put more control into the system to ensure that it is both beneficial to people and to the environment.
More information: Dimitris M. Vlachogiannis et al, HumanLight: Incentivizing ridesharing via human-centric deep reinforcement learning in traffic signal control, Transportation Research Part C: Emerging Technologies (2024). DOI: 10.1016/j.trc.2024.104593
Citation: Q&A: Researchers discuss using AI to encourage carpooling and shared transportation (2024, June 13) retrieved 13 June 2024 from https://techxplore.com/news/2024-06-qa-discuss-ai-carpooling.html
This document is subject to copyright. Apart from any fair dealing for the purpose of private study or research, no part may be reproduced without the written permission. The content is provided for information purposes only.