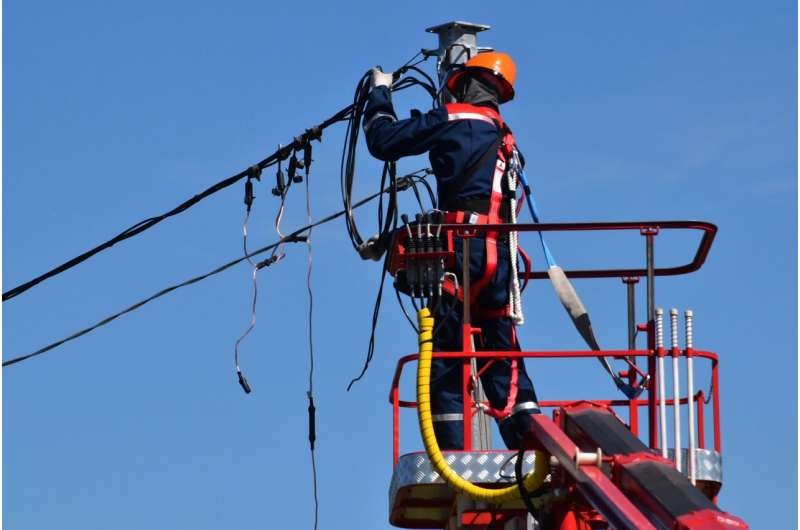
Unplanned power outages due to environmental conditions (wind, lightning, tree growth, etc.) leave those without power on their own, sometimes for long periods of time. Utility companies lack the ability to predict when forced outages will occur, so no mitigation measures targeting consumers are deployed ahead of time to reduce the impact of an outage.
Dr. Mladen Kezunovic, a professor in the Department of Electrical and Computer Engineering at Texas A&M University, and his team are combining historical outage data and weather-related data, often called big data, and machine learning to predict outages and change the outage mitigation paradigm from reactive to proactive. This will help consumers be prepared.
Using machine learning and a variety of data describing the causes of outages, the team can study data from the past to make predictions about the future.
"A lot of people ask how you test something that has never been done before," Kezunovic said. "Say you're making predictions using data from the past. You're not predicting what will happen in the summer of 2024, you are predicting what actually happened in the summer of 2023, and then comparing what happened in 2023 versus what you predicted would happen. If you were correct about the past, it should work in the future."
Once they gather the necessary data, they can superimpose database models on physics-based models to predict the state of risk of an outage.
"Wind, precipitation and lightning can all cause an outage," Kezunovic said. "We're dealing with over 60 different parameters like precipitation, temperature, wind, soil type, vegetation type, and animal intrusion from different databases that must be correlated. There is no human cognitive ability to correlate that manually, but machines can."
They correlate the risk of an outage represented by a data model with the physical disposition of the transmission lines and feeders—wires that go from substations to buildings and houses using geographic information systems (GIS). GIS involves overlaying information—in this case predictions based on models—onto geographically dispersed elements such as a grid.
"If there's rain and lighting on a specific feeder, the feeder is our physical model, then the risk of outage may occur on a section of a feeder, and because the feeder connects to the homes, these may be without electricity," Kezunovic said.
All this information is then compiled into one collapsible model that depicts the state of risk of an outage in space and time. The team can layer variables such as the ground, grid, vegetation, lightning, rain and wind like a cake. Once the model looks at the data, it can predict which area may be at risk for an outage and inform consumers in that area. The team is currently working on packaging specific communication messages for different consumer types.
The team is also educating children and young adults about power outages. They are working closely with The DoSeum, a museum for kids in San Antonio, to teach young children about what an outage is and what to do when faced with the loss of power to increase awareness and reduce panic.
The team is working with The Franklin Institute in Philadelphia to teach high school students how to predict weather and temperature so that students can further develop their STEM skills. For college students, the team plans to develop a senior capstone project around smartphone applications that alert consumers about outages and what mitigation measures to pursue to reduce the outage impact.
"For instance, as a receiver of the alert message you could describe yourself in the app, and it could pull up options to reduce the impact," Kezunovic said.
"It could tell you not to open the refrigerator because the thermal capacity of the refrigerator is to hold the cold in for 'x' many hours. Students are innovative; they may come up with some ways of telling you certain things in a neat way. Outreach is important to us, from young kids to high schoolers to college seniors to start learning about energy and power."
Other collaborators include Dr. Alexander Brown from the Department of Economics at Texas A&M, Dr. Zoran Obradovic from Temple University, Dr. Roger Enriquez from The University of Texas at San Antonio and Dr. Paul Pavlou from the University of Houston. The entire team is backed by the National Science Foundation through a project titled, "Advanced Learning for Energy Risk Tracking (ALERT)." The team also includes several graduate students from Texas A&M and Temple University.
"With artificial intelligence as a theoretical framework, you can move across disciplines," Kezunovic said.
"Dr. Obradovic has expertise in biomedical applications of AI, but his techniques are applicable to what we do. If you have data and predictions, the mathematical approach is the same, only the data is different."
Citation: Combining big data and machine learning to predict power outages and help consumers prepare (2024, March 4) retrieved 4 March 2024 from https://techxplore.com/news/2024-03-combining-big-machine-power-outages.html
This document is subject to copyright. Apart from any fair dealing for the purpose of private study or research, no part may be reproduced without the written permission. The content is provided for information purposes only.