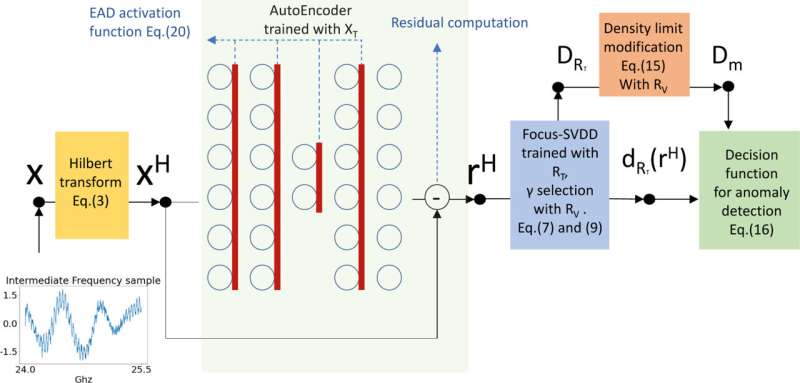
An international collaboration between EPFL and the University of Glasgow has led to an advanced machine-learning algorithm to effectively detect concealed manufacturing defects in wind turbine composite blades—before turbines are put into service.
Faulty wind turbine blades can incur huge costs for the companies that operate them, especially if the defects go unnoticed until it's too late. That's why quality assurance is such a strategic issue for global wind turbine manufacturers. Today, quality inspections are limited to surface inspection of limited areas as these composite structures roll off the production line.
But under a new approach co-created by EPFL and University of Glasgow researchers, inspection engineers can use a new patented radar technology, combined with an AI assistant, to detect possible anomalies beneath the surface. This approach has many advantages: it's non-destructive, non-contact, supports agile and rapid data acquisition and analysis, and requires very little power to operate. The research has just been published in Mechanical Systems and Signal Processing.
Merging signal processing and AI
The research draws on earlier work from both institutional partners. This work was led by Olga Fink, today a tenure-track assistant professor of civil engineering and head of the Intelligent Maintenance and Operations Systems Laboratory (IMOS) within EPFL's School of Architecture, Civil and Environmental Engineering (ENAC).
In previous research, she has developed methods for detecting anomalies by processing the sounds produced by faulty machines, for background noise suppression on audio recordings and for classifying bird songs by infusing learning capabilities into well-known and well-grounded signal processing approaches.
"Manufacturers are building wind turbines bigger, with more complicated designs. All that increases the chances of a defect occurring during the manufacturing stage," says Olga Fink, head of the Intelligent Maintenance and Operations Systems Laboratory (IMOS).
Today, she's looking at new applications for her AI-driven systems. "Wind turbines are made from several different composite materials like fiberglass and carbon fiber," she says. "Manufacturers are also building them bigger, with more complicated designs. All that increases the chances of a defect occurring during the manufacturing stage."
Measurement technology
The University of Glasgow team, led by Prof. David Flynn, James Watt School of Engineering, and Head of Research Division for Autonomous Systems and Connectivity has pioneered methods in prognostics and health management. They have explored how Robotics and Artificial Intelligence (RAI) can support net zero infrastructure.
The researchers in Glasgow used a patented Frequency Modulated Continuous Wave radar with a robotic arm to inspect industrial wind-turbine blade samples at distances of 5, 10 and 15 centimeters from the sample. Using signal processing methods, they could isolate features and precursors to future failures in these complex composite samples (see below a video produced by the University of Glasgow).
More information: Gaëtan Frusque et al, Non-contact sensing for anomaly detection in wind turbine blades: A focus-SVDD with complex-valued auto-encoder approach, Mechanical Systems and Signal Processing (2023). DOI: 10.1016/j.ymssp.2023.111022
Citation: AI-driven method helps improve quality assurance for wind turbines (2024, February 22) retrieved 22 February 2024 from https://techxplore.com/news/2024-02-ai-driven-method-quality-turbines.html
This document is subject to copyright. Apart from any fair dealing for the purpose of private study or research, no part may be reproduced without the written permission. The content is provided for information purposes only.