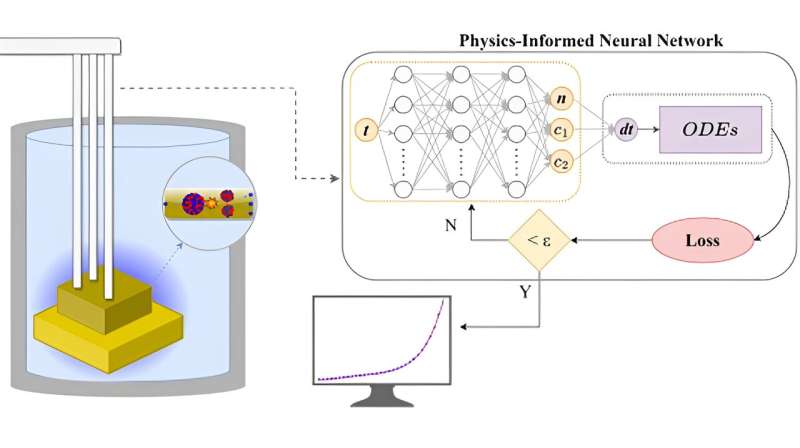
American nuclear power plants generate more than 20% of the electricity, and half of the carbon-free electricity, in the United States. The nation's pressing demand for even more electricity—specifically carbon-free electricity that does not contribute to climate change—is spurring interest in advanced nuclear technologies. It is also leading many to ask if the nuclear power industry, sometimes labeled more expensive to maintain and operate than other clean energy industries, can modernize itself and present a more compelling bottom line.
Alex Heifetz, principal nuclear engineer at the U.S. Department of Energy's (DOE) Argonne National Laboratory, thinks new artificial intelligence and machine learning tools and techniques will pave a path to "yes."
Heifetz and a team of researchers used PUR-1, Purdue University's NRC-commissioned, all-digital research reactor, to conduct a series of experiments using a physics-informed neural network with transfer learning (TL-PINN). Neural networks are methods within machine learning that can be trained to recognize patterns in data. However, they are not great at making predictions outside of their training domain. Researchers like Heifetz believe PINNs can overcome this shortcoming by fusing neural networks with differential equations to solve complex scientific or engineering problems. This would help them extrapolate to new scenarios and make more accurate predictions.
In work published in Nature's Scientific Reports, Heifetz's team created a model of a small modular reactor (SMR) with automated operations and then used machine learning to do something the nuclear industry would never attempt in an operating nuclear power plant: they tinkered with its data and training models.
"We focused on the specific issue of algorithm performance," said Heifetz. "The more data you have, the better your model becomes in terms of prediction accuracy. With newer technologies and designs, you may not have all the data handy to make a prediction. We applied the concept of transfer learning, in which you retrain the PINN with a synthetic data set and then you look at the similarity between the data sets to see if you can rely on the synthetic data to make accurate predictions."
Many previous studies have focused on the safety and security of nuclear reactors, but this research narrowed its lens specifically on cost. The overall objective, Heifetz said, is to reduce operations and maintenance costs and enable development and commercialization of low-cost SMRs. Small modular reactors produce 300 megawatts of power or less, compared to current reactors, which have power ratings of about 1,000 megawatts (enough power to provide electricity to approximately 1,000,000 homes).
Many think SMRs could vastly expand the impact of nuclear energy. They take up less space than existing nuclear reactors, are less expensive to build, operate and maintain, and have modern features such as digital gauges, devices and sensors, which most existing reactors did not originally have. They can be used to provide power in remote parts of the United States, or possibly in futuristic waystations that make electrification of long-haul trucking more plausible.
"Building a new nuclear reactor is difficult so we don't want to shut down the ones we have," said Heifetz. "The challenge is to take a $10 billion nuclear reactor that was built in the 1950s and—while preserving all its safety features—make it more modern, efficient and autonomous, with fewer operators and less shut-down time. But, we also want to test new designs, like SMRs, for which there isn't enough data. By developing a physics-informed neural network that doesn't require much time to train, maybe we can lower the cost of training new models."
More information: Konstantinos Prantikos et al, Physics-informed neural network with transfer learning (TL-PINN) based on domain similarity measure for prediction of nuclear reactor transients, Scientific Reports (2023). DOI: 10.1038/s41598-023-43325-1
Citation: What if American nuclear power plants could be less expensive (2024, January 26) retrieved 26 January 2024 from https://techxplore.com/news/2024-01-american-nuclear-power-expensive.html
This document is subject to copyright. Apart from any fair dealing for the purpose of private study or research, no part may be reproduced without the written permission. The content is provided for information purposes only.