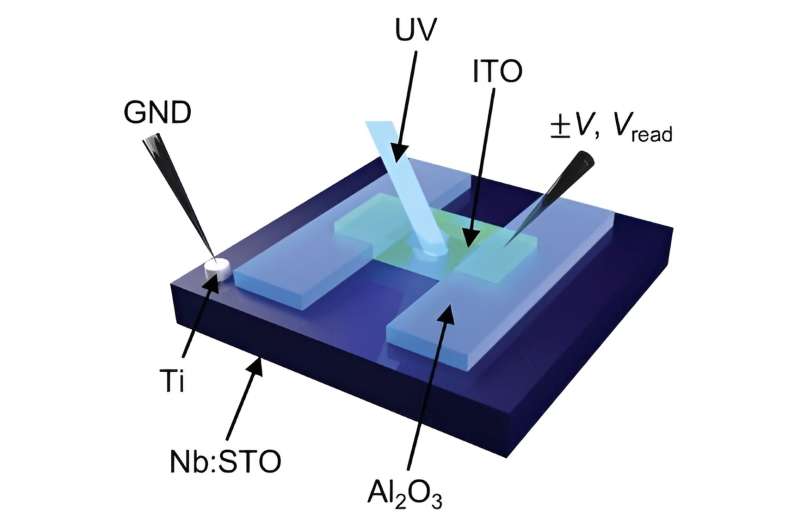
Every day, a significant amount of data related to weather, traffic, and social media undergo real-time processing. In traditional cloud computing, this processing occurs on the cloud, raising concerns about issues such as leaks, communication delays, slow speeds, and higher power consumption.
Against this backdrop, "edge computing" presents a promising alternative solution. Located near users, it aims to distribute computations, thereby reducing the load and speeding up data processing. Specifically, edge AI, which involves AI processing at the edge, is expected to find applications in, for example, self-driving cars and machine anomaly prediction in factories.
However, for effective edge computing, efficient and computationally cost-effective technology is needed. One promising option is reservoir computing, a computational method designed for processing signals that are recorded over time. It can transform these signals into complex patterns using reservoirs that respond nonlinearly to them.
In particular, physical reservoirs, which use the dynamics of physical systems, are both computationally cost-effective and efficient. However, their ability to process signals in real time is limited by the natural relaxation time of the physical system. This limits real-time processing and requires adjustments for best learning performance.
Recently, Professor Kentaro Kinoshita, a member of the Faculty of Advanced Engineering and the Department of Applied Physics at the Tokyo University of Science (TUS), and Mr. Yutaro Yamazaki from the Graduate School of Science and the same department at TUS developed an optical device with features that support physical reservoir computing and allow real-time signal processing across a broad range of timescales within a single device. Their findings were published in Advanced Science on 20 November 2023.
Speaking of their motivation for the study, Prof. Kinoshita explains, "The devices developed in this research will enable a single device to process time-series signals with various timescales generated in our living environment in real time. In particular, we hope to realize an AI device to utilize in the edge domain."
In their study, the duo created a special device using Sn-doped In2O3 and Nb-doped SrTiO3 (denoted as ITO/Nb:STO), which responds to both electrical and optical signals. They tested the electrical features of the device to confirm that it functions as a memristor (a memory device that can change its electrical resistance). The team also explored the influence of ultraviolet light on ITO/Nb:STO by varying the voltage and observing changes in the current. The results suggested that this device can modify the relaxation time of the photo-induced current according to the voltage, making it a potential candidate for a physical reservoir.
Furthermore, the team tested the effectiveness of ITO/Nb:STO as a physical reservoir by using it for classifying handwritten digit images in the MNIST (Modified National Institute of Standards and Technology) dataset. To their delight, the device achieved a classification accuracy of up to 90.2%. Additionally, to understand the role of the physical reservoir, the team ran experiments without it, which resulted in a relatively lower classification accuracy of 85.1%. These findings show that the ITO/Nb:STO junction device improves classification accuracy while keeping computational costs lower, proving its value as a physical reservoir.
"In the past, our research group has focused on research and development of materials applicable to physical reservoir computing. Accordingly, we fabricated these devices with the aim to realize a physical reservoir in which the relaxation time of photo-induced current can be arbitrarily controlled by voltage," says Prof. Kinoshita.
In summary, this study presents a novel memristor device capable of adjusting its response timescale through voltage variation, exhibiting enhanced learning capabilities, which makes it promising for applications at the edge as an AI device for edge computing. This, in turn, could pave the way for single devices that can effectively handle signals of varied durations found in real-world environments.
More information: Yutaro Yamazaki et al, Photonic Physical Reservoir Computing with Tunable Relaxation Time Constant, Advanced Science (2023). DOI: 10.1002/advs.202304804
Citation: Revolutionizing real-time data processing with edge computing and reservoir technology (2024, January 11) retrieved 11 January 2024 from https://techxplore.com/news/2024-01-revolutionizing-real-edge-reservoir-technology.html
This document is subject to copyright. Apart from any fair dealing for the purpose of private study or research, no part may be reproduced without the written permission. The content is provided for information purposes only.