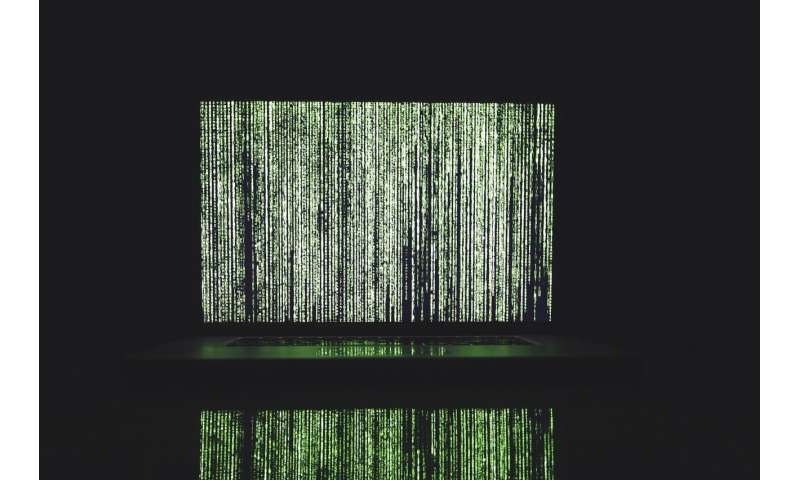
In a study with potentially far-reaching implications for criminal justice in the United States, a team of California researchers has found that algorithms are significantly more accurate than humans in predicting which defendants will later be arrested for a new crime.
When assessing just a handful of variables in a controlled environment, even untrained humans can match the predictive skill of sophisticated risk-assessment instruments, says the new study by scholars at Stanford University and the University of California, Berkeley.
But real-world criminal justice settings are often far more complex, and when a larger number of factors are useful for predicting recidivism, the algorithm-based tools performed far better than people. In some tests, the tools approached 90% accuracy in predicting which defendants might be arrested again, compared to about 60% for human prediction.
"Risk assessment has long been a part of decision-making in the criminal justice system," said Jennifer Skeem, a psychologist who specializes in criminal justice at UC Berkeley. "Although recent debate has raised important questions about algorithm-based tools, our research shows that in contexts resembling real criminal justice settings, risk assessments are often more accurate than human judgment in predicting recidivism. That's consistent with a long line of research comparing humans to statistical tools."
"Validated risk-assessment instruments can help justice professionals make more informed decisions," said Sharad Goel, a computational social scientist at Stanford University. "For example, these tools can help judges identify and potentially release people who pose little risk to public safety. But, like any tools, risk assessment instruments must be coupled with sound policy and human oversight to support fair and effective criminal justice reform."
The paper—"The limits of human predictions of recidivism"—was slated for publication Feb. 14, 2020, in Science Advances. Skeem presented the research on Feb. 13 in a news briefing at the annual meeting of the American Association for the Advancement of Science (AAAS) in Seattle, Wash. Joining her were two co-authors: Ph.D. graduate Jongbin Jung and Ph.D. candidate Zhiyuan "Jerry" Lin, who both studied computational social science at Stanford.
The research findings are important as the United States debates how to balance the needs communities have for security while reducing incarceration rates that are the highest of any nation in the world—and disproportionately affect African Americans and communities of color.
If the use of advanced risk assessment tools continues and improves, that could refine critically important decisions that justice professionals make daily: Which individuals can be rehabilitated in the community, rather than in prison? Which could go to low-security prisons, and which to high-security sites? And which prisoners can safely be released to the community on parole?
Assessment tools driven by algorithms are widely used in the United States, in areas as diverse as medical care, banking and university admissions. They have long been used in criminal justice, helping judges and others to weigh data in making their decisions.
But in 2018, researchers at Dartmouth University raised questions about the accuracy of such tools in a criminal justice framework. In a study, they assembled 1,000 short vignettes of criminal defendants, with information drawn from a widely used risk assessment called the Correctional Offender Management Profiling for Alternative Sanctions (COMPAS).
The vignettes each included five risk factors for recidivism: the individual's sex, age, current criminal charge, and the number of previous adult and juvenile offenses. The researchers then used Amazon's Mechanical Turk platform to recruit 400 volunteers to read the vignettes and assess whether each defendant would commit another crime within two years. After reviewing each vignette, the volunteers were told whether their evaluation accurately predicted the subject's recidivism.
Both the people and the algorithm were accurate slightly less than two-thirds of the time.
These results, the Dartmouth authors concluded, cast doubt on the value of risk-assessment instruments and algorithmic prediction.
The study generated high-profile news coverage—and sent a wave of doubt through the U.S. criminal justice reform community. If sophisticated tools were no better than people in predicting which defendants would re-offend, some said, then there was little point in using the algorithms, which might only reinforce racial bias in sentencing. Some argued such profound decisions should be made by people, not computers.
Grappling with "noise" in complex decisions
But when the authors of the new California study evaluated additional data sets and more factors, they concluded that that risk assessment tools can be much more accurate than people in assessing potential for recidivism.
The study replicated the Dartmouth findings that had been based on a limited number of factors. However, the information available in justice settings is far more rich—and often more ambiguous.
"Pre-sentence investigation reports, attorney and victim impact statements, and an individual's demeanor all add complex, inconsistent, risk-irrelevant, and potentially biasing information," the new study explains.
The authors' hypothesis: If research evaluations operate in a real-world framework, where risk-related information is complex and "noisy," then advanced risk assessment tools would be more effective than humans at predicting which criminals would re-offend.
To test the hypothesis, they expanded their study beyond COMPAS to include other data sets. In addition to the five risk factors used in the Dartmouth study, they added 10 more, including employment status, substance use and mental health. They also expanded the methodology: Unlike the Dartmouth study, in some cases the volunteers would not be told after each evaluation whether their predictions were accurate. Such feedback is not available to judges and others in the court system.
The outcome: Humans performed "consistently worse" than the risk assessment tool on complex cases when they didn't have immediate feedback to guide future decisions.
For example, the COMPAS correctly predicted recidivism 89% of the time, compared to 60% for humans who were not provided case-by-case feedback on their decisions. When multiple risk factors were provided and predictive, another risk assessment tool accurately predicted recidivism over 80% of the time, compared to less than 60% for humans.
The findings appear to support continued use and future improvement of risk assessment algorithms. But, as Skeem noted, these tools typically have a support role. Ultimate authority rests with judges, probation officers, clinicians, parole commissioners and others who shape decisions in the criminal justice system.
More information: Z. Lin el al., "The limits of human predictions of recidivism," Science Advances (2020). DOI: 10.1126/scaidv.aaz0652 , https://advances.sciencemag.org/content/6/7/eaaz0652
Citation: Algorithms 'consistently' more accurate than people in predicting recidivism, study says (2020, February 14) retrieved 14 February 2020 from https://techxplore.com/news/2020-02-algorithms-accurate-people-recidivism.html
This document is subject to copyright. Apart from any fair dealing for the purpose of private study or research, no part may be reproduced without the written permission. The content is provided for information purposes only.