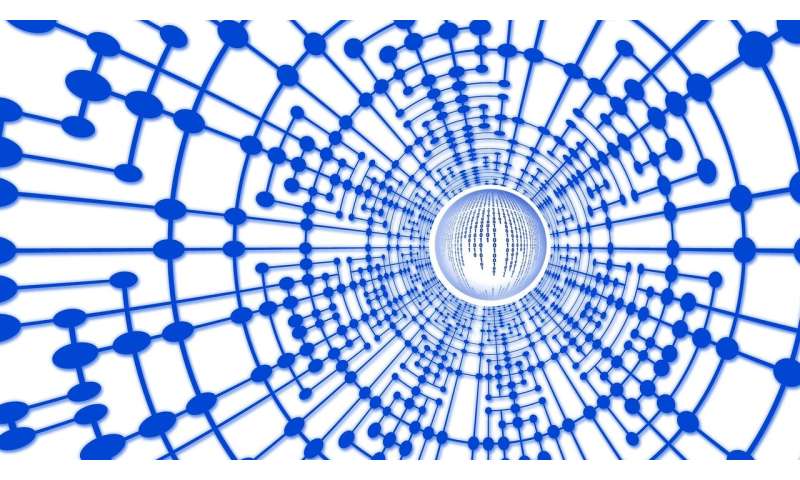
A combined team of researchers from Babylon Health and University College has created an algorithm that they claim can find causal relationships among information in overlapping medical datasets. They have written a paper describing their algorithm and have uploaded it to the arXiv preprint server. They will also be giving a presentation describing their research at this year's Association for Advancement of Artificial Intelligence meeting.
Finding a systematic way to sift through data to find the cause of a given condition in a single sick person is a major challenge in AI research. If a patient has been sneezing more than normal lately, is it because an allergen has been introduced into their environment? Or have they caught a cold? Worse, maybe they have a cancerous tumor in their sinuses or brain. The current system for seeking the right answer in such scenarios is human-based. Doctors ask questions and search their memory for answers. If they are unable to find one, they may consult with other doctors or study medical textbooks or online databases.
This system has its merits, of course, it being the best available. But it also has drawbacks—it is limited by human memory and resourcefulness. Many computer specialists believe there is a better way—let a computer do it. This is not currently possible, but scientists are working on it. In this new effort, the researchers have introduced a system with an algorithm that analyzes data from disparate, overlapping datasets and finds causal relationships.
The algorithm is based on the concept of entropy, in which any system becomes more disordered over time. The researchers propose that entropy exists with information in datasets, as well, and that causal forces are more ordered than the data that describes the outcome of their effects. That being the case, it should be possible to work backward to find the cause—and that is just what their algorithm does.
The system was able to correctly assess the size and texture of breast cancer tumors when comparing datasets in which the causal relationships were already known—it determined that they did not have a causal link, but both were an indicator of whether a tumor was benign or malignant.
More information: Anish Dhir, Ciarán M. Lee. Integrating overlapping datasets using bivariate causal discovery. arXiv:1910.11356v2 [stat.ML]: arxiv.org/abs/1910.11356
© 2020 Science X Network
Citation: Using AI to spot causative relationships in overlapping medical datasets (2020, February 7) retrieved 7 February 2020 from https://techxplore.com/news/2020-02-ai-relationships-overlapping-medical-datasets.html
This document is subject to copyright. Apart from any fair dealing for the purpose of private study or research, no part may be reproduced without the written permission. The content is provided for information purposes only.